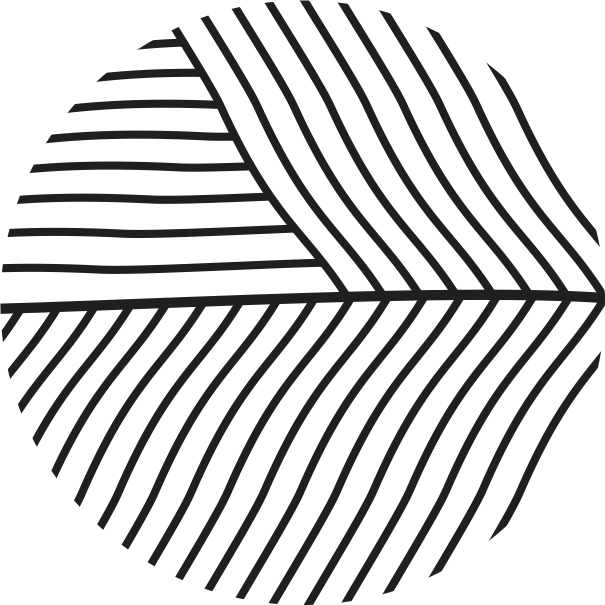
The Igarapé Institute uses cookies and other similar technologies to improve your experience, in accordance with our Privacy Policy and our Terms of Use, and by continuing to browse, you agree to these conditions.
public version
crimeradar@igarape.org.br
The Igarapé Institute uses cookies and other similar technologies to improve your experience, in accordance with our Privacy Policy and our Terms of Use, and by continuing to browse, you agree to these conditions.
O Instituto Igarapé utiliza cookies e outras tecnologias semelhantes para melhorar a sua experiência, de acordo com a nossa Política de Privacidade e nossos Termos de Uso e, ao continuar navegando, você concorda com essas condições.